AI/ML
Retrieval Augmented Generation (RAG) Pipelines
Retrieval Augmented Generation (RAG) pipelines are a crucial component of generative AI, enhancing the model's ability to generate accurate and contextually relevant content. RAG pipelines operate through a streamlined process involving data preparation, data retrieval, and response generation.
- Phase 1: Data Preparation
During the data preparation phase, raw data such as text, audio, etc., is extracted and divided into smaller chunks. These chunks are then translated into embeddings and stored in a vector database. It is important to store the chunks and their metadata together with the embeddings in order to reference back to the actual source of information in the retrieval phase. - Phase 2: Data Retrieval
The retrieval phase is initiated by a user prompt or question. An embedding of this prompt is created and used to search for the most similar pieces of content in the vector database. The relevant data extracted from the source data is used as context, along with the original question, for the Large Language Model (LLM) to generate a response.
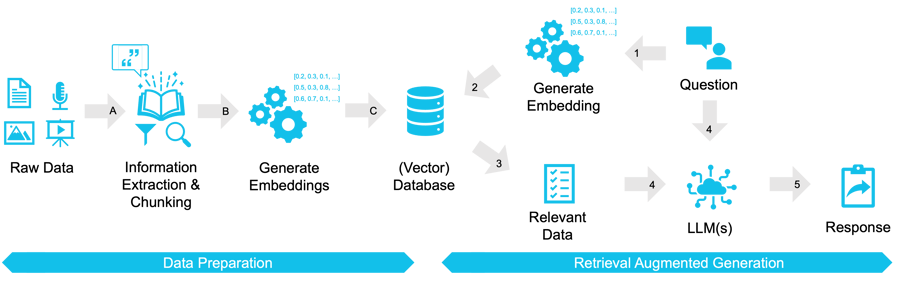