TGW Logistics Group is one of the leading international suppliers of material handling solutions. For more than 50 years, the Austrian specialist has implemented automated systems for its international customers, including brands from A as in Adidas to Z as in Zalando. As systems integrator, TGW plans, produces and implements complex logistics centres, from mechatronic products and robots to control systems and software.
The use case for TGW is to expedite the aggregation and access of large amounts of varied data collected in real-time from warehouse systems worldwide. Their warehouse solutions typically consist of the shuttle engine (the actual warehouse), a conveyor network, and pick centers where goods are packaged for shipping.
The Digital Twin of the warehouses is used to offer Digital Assistants in the following ways:
Process Monitoring:
- Implements data-driven and AI-based supervision of logistic processes that constantly monitors warehouse operations.
- Identifies anomalies in the processes, such as a decrease in picking performance.
- Notifies the operator when actions need to be taken to rectify the anomalies.
- Recommends actions to remove the identified anomalies
Example: Anomaly Detection in a Pickcenter
- Anomalies can arise from the interruption in the supply of source totes, affecting picking performance.
- Interruption in the supply of target totes can also be an anomaly.
- The presence of a slow picker or an unplanned picker break can also lead to decreased performance.
Example: Recommendations for Pickcenter
- The system can recommend opening another pickcenter based on different order profiles and shipping times.
- This ensures that all shipping times are met and there are no delays in order delivery.
- For instance, if an error causes the warehouse to stand still and miss the shipping time, it can affect the order delivery for the clients.
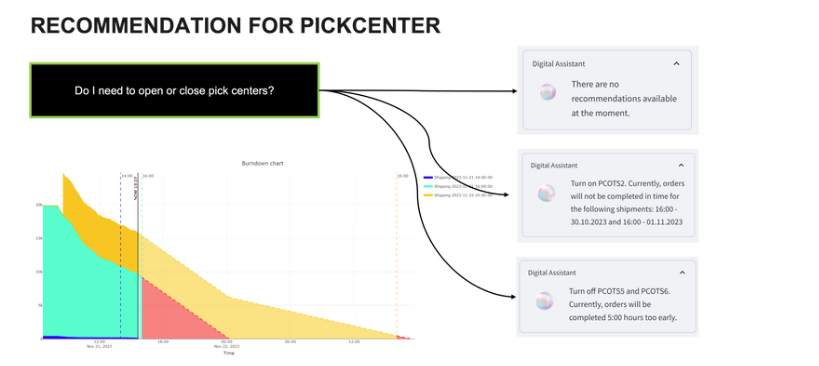
Looking ahead, TGW envisions a further combination of these diverse datasets. The ultimate goal is to provide an agent-based assistant for their end-customers, designed to navigate the various machine learning solutions, extract solutions, reason, and ultimately suggest an appropriate solution to a problem. This is seen as the future direction in the industrial context, where the complexity of systems and the need for domain knowledge require such advanced, data-driven solutions.