Time Series Data
Time series data querying with SQL
Hyper-fast. Results in milliseconds.
/* Based on device data, this query returns the average
* of the battery level for every hour for each device_id
*/
WITH avg_metrics AS (
SELECT device_id,
DATE_BIN('1 hour'::INTERVAL, time, 0) AS period,
AVG(battery_level) AS avg_battery_level
FROM devices.readings
GROUP BY 1, 2
ORDER BY 1, 2
)
SELECT period,
t.device_id,
manufacturer,
avg_battery_level
FROM avg_metrics t, devices.info i
WHERE t.device_id = i.device_id
AND model = 'mustang'
LIMIT 10;
+---------------+------------+--------------+-------------------+
| period | device_id | manufacturer | avg_battery_level |
+---------------+------------+--------------+-------------------+
| 1480802400000 | demo000001 | iobeam | 49.25757575757576 |
| 1480806000000 | demo000001 | iobeam | 47.375 |
| 1480802400000 | demo000007 | iobeam | 25.53030303030303 |
| 1480806000000 | demo000007 | iobeam | 58.5 |
| 1480802400000 | demo000010 | iobeam | 34.90909090909091 |
| 1480806000000 | demo000010 | iobeam | 32.4 |
| 1480802400000 | demo000016 | iobeam | 36.06060606060606 |
| 1480806000000 | demo000016 | iobeam | 35.45 |
| 1480802400000 | demo000025 | iobeam | 12 |
| 1480806000000 | demo000025 | iobeam | 16.475 |
+---------------+------------+--------------+-------------------+
/* To identify gaps on the readings, the following queries generates a series
* and by joining it with the original data, you can spot any gap */
with avg_battery AS (
SELECT battery_level, time
FROM devices.readings
WHERE device_id = 'demo000007'
AND time > 1480118400000
AND time < 1480301200000
ORDER BY 2
),
all_hours AS (
SELECT generate_series(1480118430000,1480301200000,'30 second'::interval) AS generated_hours
)
SELECT time, generated_hours, battery_level
FROM all_hours
LEFT JOIN avg_battery ON generated_hours = time
ORDER BY 2
LIMIT 20;
+---------------+---------------+---------------+
| time | hours | battery_level |
+---------------+---------------+---------------+
| 1480118430000 | 1480118430000 | 67 |
| 1480118460000 | 1480118460000 | 66 |
| 1480118490000 | 1480118490000 | 66 |
| 1480118520000 | 1480118520000 | 66 |
| 1480118550000 | 1480118550000 | 66 |
| 1480118580000 | 1480118580000 | 66 |
| 1480118610000 | 1480118610000 | 65 |
| 1480118640000 | 1480118640000 | NULL |
| 1480118670000 | 1480118670000 | 65 |
| 1480118700000 | 1480118700000 | 65 |
| 1480118730000 | 1480118730000 | 65 |
| 1480118760000 | 1480118760000 | 65 |
| 1480118790000 | 1480118790000 | 65 |
| 1480118820000 | 1480118820000 | 65 |
| 1480118850000 | 1480118850000 | 65 |
| 1480118880000 | 1480118880000 | 65 |
| 1480118910000 | 1480118910000 | 65 |
| 1480118940000 | 1480118940000 | 65 |
| 1480118970000 | 1480118970000 | NULL |
| 1480119000000 | 1480119000000 | NULL |
+---------------+---------------+---------------+
/* Based on device data, this query returns the number of battery charges
* per day for a given device_id */
WITH aux_charging AS (
SELECT time,
DATE_BIN('P1D'::INTERVAL,time,0) AS day,
battery_status,
LAG(battery_status) OVER (PARTITION BY device_id ORDER BY time) AS prev_battery_status
FROM devices.readings
WHERE device_id = 'demo000001'
ORDER BY time
),
count_start_charging AS (
SELECT day, (case when battery_status <> prev_battery_status then 1 else 0 end) AS start_charging
FROM aux_charging
ORDER BY 1
)
SELECT day, sum(start_charging) as charges_number
FROM count_start_charging
GROUP BY 1
ORDER BY 1;
+---------------+---------------+
| count_charges | day |
+---------------+---------------+
| 2 | 1479168000000 |
| 4 | 1479254400000 |
| 2 | 1479340800000 |
| 10 | 1479427200000 |
| 7 | 1479600000000 |
| 8 | 1479686400000 |
| 6 | 1479772800000 |
| 11 | 1479859200000 |
| 5 | 1480032000000 |
| 7 | 1480118400000 |
| 6 | 1480204800000 |
| 10 | 1480291200000 |
| 3 | 1480464000000 |
| 3 | 1480550400000 |
| 7 | 1480636800000 |
| 2 | 1480723200000 |
+---------------+---------------+
/* Based on device data, this query returns the average of the battery temperature
* for each OS version */
SELECT device_info['os_name'], avg(battery_temperature)
FROM "devices"."readings"
GROUP BY 1
LIMIT 100;
+---------+--------------------------+
| os_name | avg(battery_temperature) |
+---------+--------------------------+
| 4.4.4 | 90.85937893039049 |
| 5.1.0 | 90.86754559738132 |
| 6.0.1 | 90.84230101265824 |
| 5.0.0 | 90.8574802739726 |
+---------+--------------------------+
Columnar storage
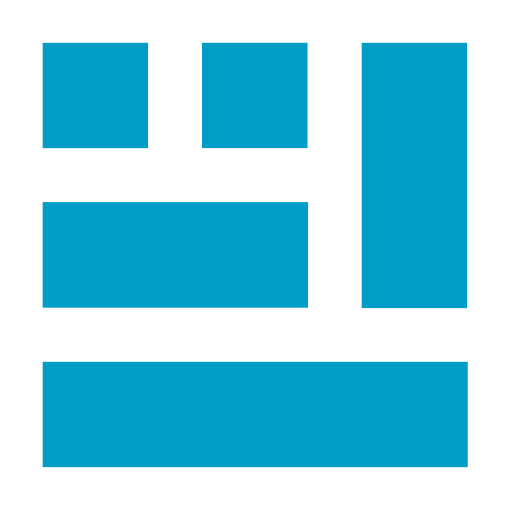
High cardinality
CrateDB offers robust support for time partitioning. It enables data to be stored long-term without any need for aggregation or down-sampling. This is crucial because the original, un-aggregated data often contains granular details that may be lost during aggregation. By preserving this level of detail, you get enhanced flexibility to revisit historical data for new insights, or conduct precise forecasting, which is crucial to strategic decision-making processes.
With CrateDB, you can also move your old partitions to slow but cheap spinning disks, while keeping the most recent data on fast SSDs, all while retaining fast query speed for most recent data, and not loosing any details in older data.
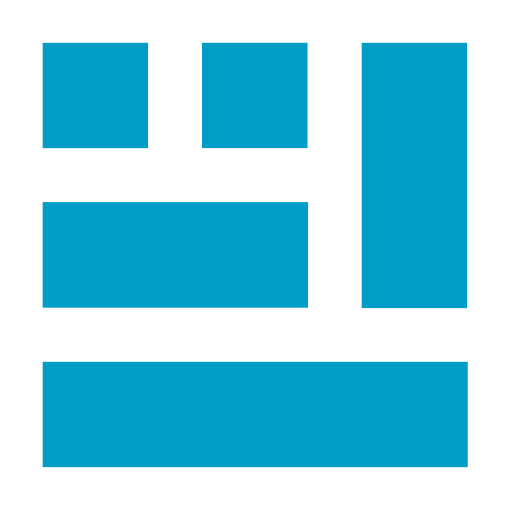
Time series functionality
CrateDB includes built-in time-series functionality, such as window functions and time-based indexes. These features make it easier to query and analyze the data, and can improve query performance.
LEAD
andLAG
functions, withIGNORE NULLS
option, to fill and extrapolate missing data.DATE_BIN
function to resample the data and use the same intervals on the time axis.WINDOW
function.JOIN
operator to easily combine time series data in one table and corresponding metadata in another table. This avoids pushing too much data in your application.
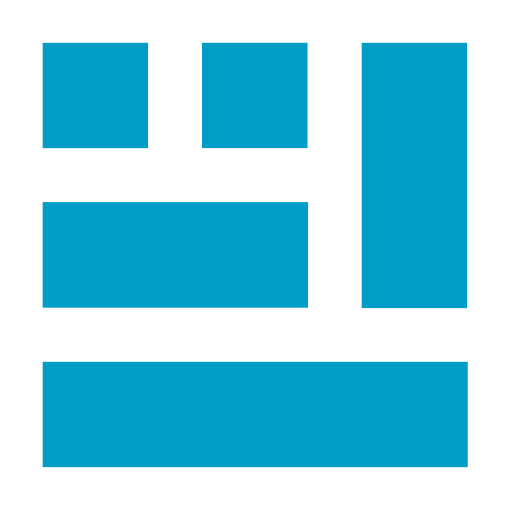
Much more than a time series database
CrateDB offers a much broader scope; it is built for multiple types of data and you can combine them all into the same database: time series, JSON, vector, full-text, geospatial, BLOB and relational. This way, you can easily cover most of your needs, without investing in some new costly technology that needs complex maintenance and data synchronization.
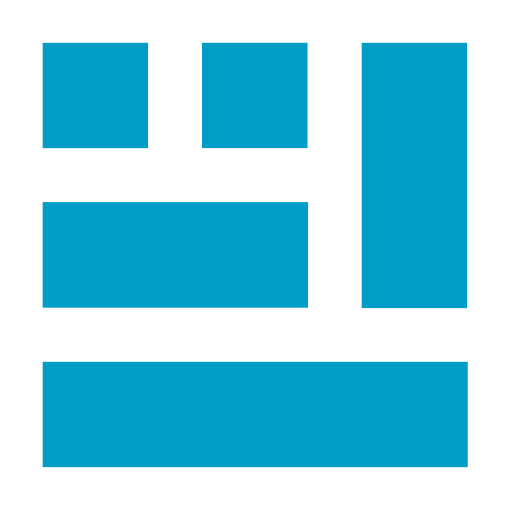
SQL interface
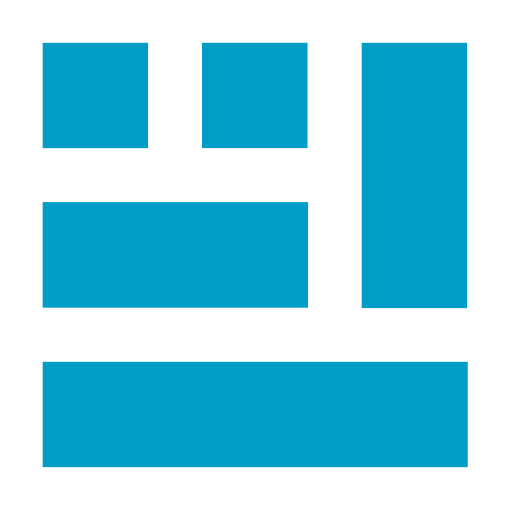
Integrations
CrateDB integrates seamlessly with popular modern data visualization tools like Grafana, or libraries like Matplotlib. It also offers compatibility with the Java- and Python-based data ecosystem and corresponding libraries and frameworks such as pandas, Dask, or Spark, to facilitate efficient analysis and visualization of time series data.
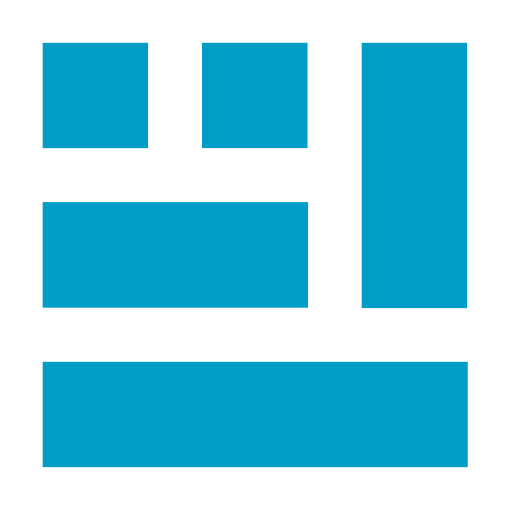
Distributed architecture
CrateDB is a distributed database that can scale horizontally across multiple nodes. This makes it an ideal fit for time series workloads, which often involve handling large volumes of data from multiple different sources (sensors, IoT gateways, CRM, ERP...) that need to be ingested, enriched and processed on the fly to serve many simultaneous data consumers in real-time.
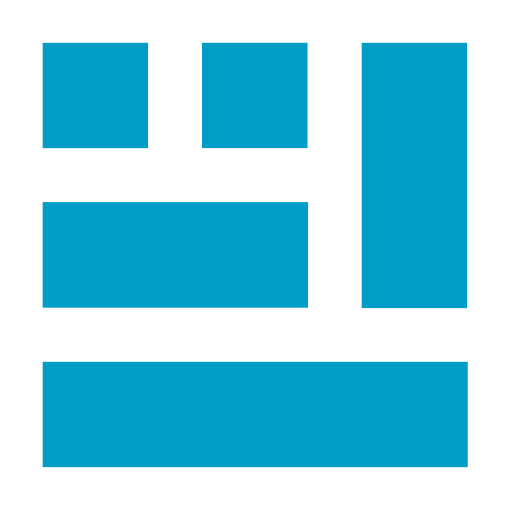
Open source
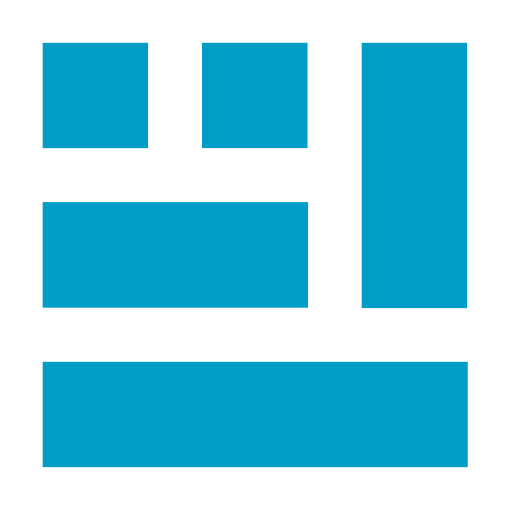
Guide for time series data projects
Guide for time series data projects
Demo: Querying heterogeneous time-series data with SQL
Demo: Querying heterogeneous time-series data with SQL
Curious to learn more?
Top 8 most asked questions
Top 8 most asked questions
Discover some of the common questions around time-series databases, including their advantages over traditional databases, best practices for managing them, and the industries that can benefit the most from their adoption. This white paper contains 8 essential things you need to know about time-series databases.
Time series online course
Time series online course
Additional resources on time series
User stories
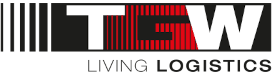
Using CrateDB, TGW accelerates data aggregation and access from warehouse systems worldwide, resulting in increased database performance. The system can handle over 100,000 messages every few seconds.
"CrateDB is a highly scalable database for time series and event data with a very fast query engine using standard SQL".
Alexander Mann
Owner Connected Warehouse Architecture
TGW Logistics Group
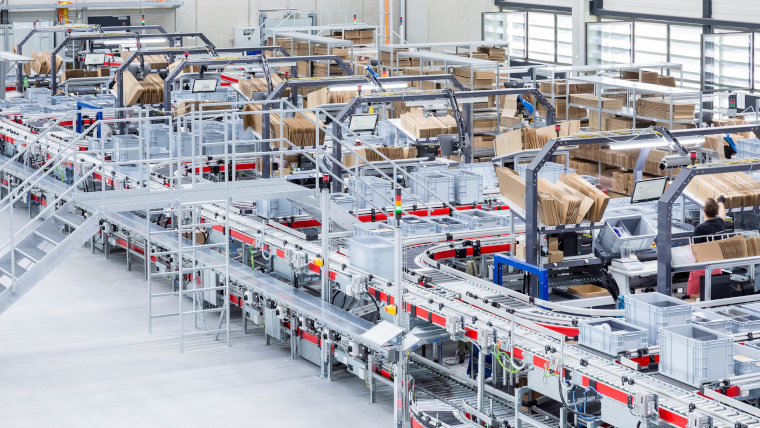
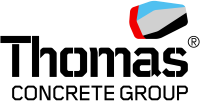
"Thanks to CrateDB's great indexing, dedicated data types, and subsequent great performance, we could execute an event and data-driven architecture, with the performance and scalability necessary for storing time-series data over time. The SQL query syntax capability of CrateDB also played a part in achieving this great outcome, as it made it easy for the team to write good performing queries using existing know-how. CrateDB is now an integral part of our big data streaming architecture and it is delivering as promised."
Kristoffer Axelsson
Principal Solution Architect
Thomas Concrete Group
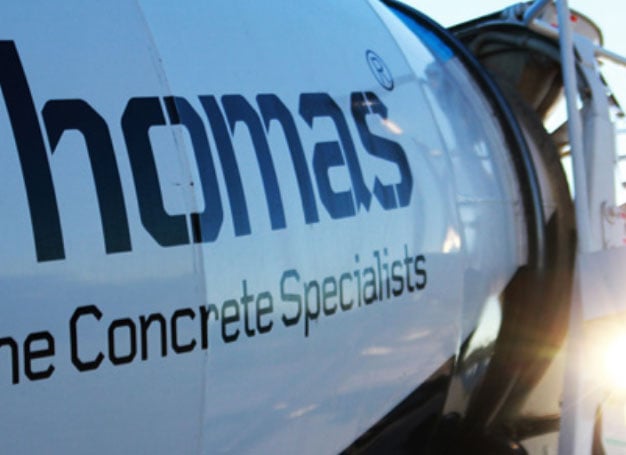

"I'm glad it's SQL behind those charts. If I had to use Elasticsearch to answer new questions, we wouldn't be nearly as responsive to new requirements."
Joe Hacobian
Infrastructure Engineer
Digital Domain
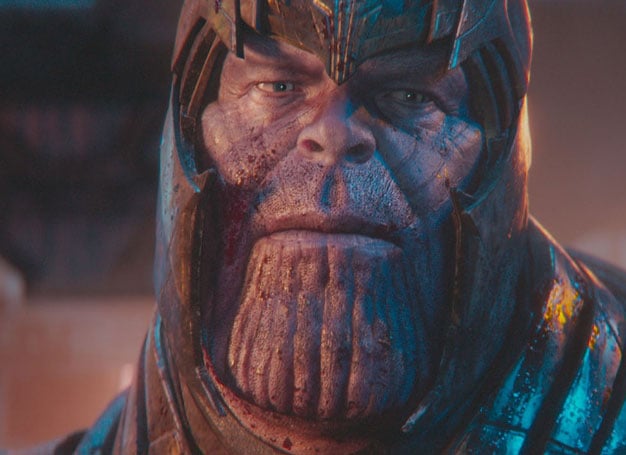
Related blog posts
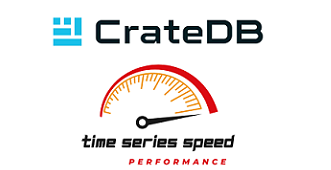
Independent Time Series Benchmark Confirms CrateDB’s Top-Tier Performance
2024-09-02CrateDB continues to deliver impressive results in the latest TSBS benchmark conducted by Nyrkio. Compared to MongoDB and InfluxDB, CrateDB excels in both ingestion capabilities and complex ad hoc ...
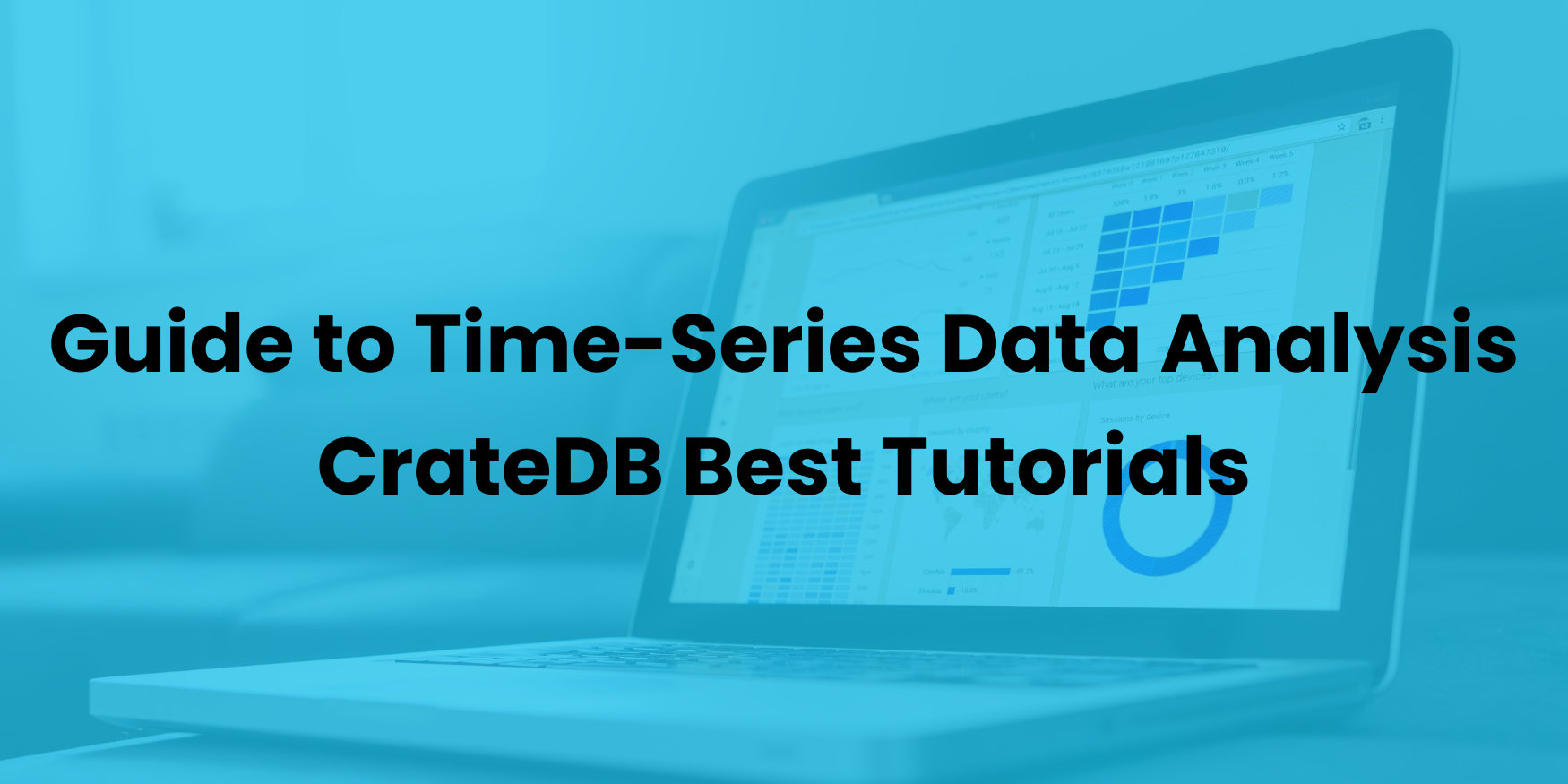
Guide to Time-Series Data Analysis in CrateDB: Best Tutorials
2023-11-29Are you looking for a way to store and analyze your time-series data efficiently? In this blog post, we'll list the best tutorials for getting started with time-series data analysis in CrateDB.
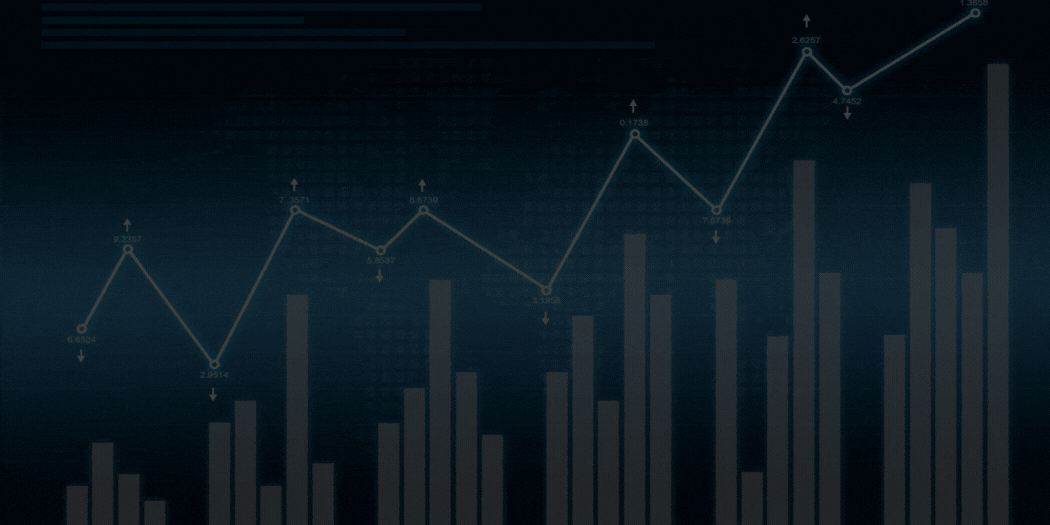
Data Historians vs Time Series Databases: A Comparative Analysis
2023-11-02Choosing your data storage solution is a critical decision for your business. How data is collected, stored, and analyzed can impact how you harness the full potential of your data, the operations, ...
Documentation and tutorials
FAQ
Time-series data is a sequence of data points organized chronologically, illustrating how variables change over time. This format is characterized by patterns such as trends, seasonal variations, and irregularities. Effective time-series data management can significantly enhance query performance and data analysis. CrateDB supports time-series data with built-in functionalities like window functions and time-based indexes, making it easier to analyze and query the data efficiently.
Examples of time series data include temperature readings, stock prices, population growth, monthly subscriptions, quarterly sales, and interest rates. CrateDB supports time series data and offers a comprehensive solution by allowing the integration of multiple data types such as JSON, vector, full-text, geospatial, BLOB, and relational data, minimizing the need for additional technologies and complex maintenance.
Efficient storage of time series data is essential, considering factors like data volume, speed, query requirements, and scalability. The primary storage solutions include time-series databases, NoSQL databases, relational databases, and data warehouses, each offering unique strengths and limitations. CrateDB is designed to handle complex time series data with the simplicity of SQL, allowing for high ingest rates and integration of various data types, making it an ideal choice for storing and querying extensive time series data efficiently. Read more about time series data storage >
When choosing a time-series database, consider factors such as performance, scalability, query language capabilities, data model flexibility, security, maintainability, and reliability. Examples of time-series databases include CrateDB, InfluxDB, KX, and Timescale. CrateDB stands out as a distributed database that can scale horizontally across multiple nodes, making it especially suited for handling large volumes of time-series data from diverse sources in real-time. Learn how to choose the best database for time series data >
101 for Time-Series databases
- What is a time series database?
- Key criteria for selecting a time series database.