AI/ML Database
Vector storage
With CrateDB's vector store, you can easily store and retrieve embeddings generated by AI and ML models, seamlessly integrating vectorized data with your existing datasets. It allows you to enrich your existing data with semantics, providing context that aligns with your data and enhancing explainability.
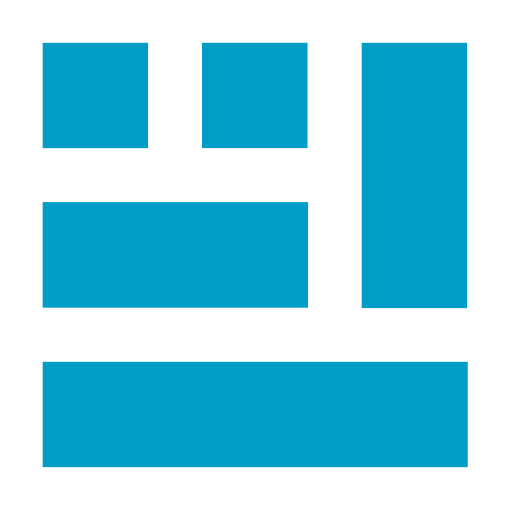
Advanced search capabilities
CrateDB offers advanced search capabilities through its similarity search and flexible filtering, combining full-text and vector search. Similarity search allows users to find similarities across any data represented as vectors, while the combination of full-text and vector search improves the search precision by enhancing semantic similarity and keyword matching. These features facilitate enhanced recommendations, anomaly detection, and other AI/ML use cases.
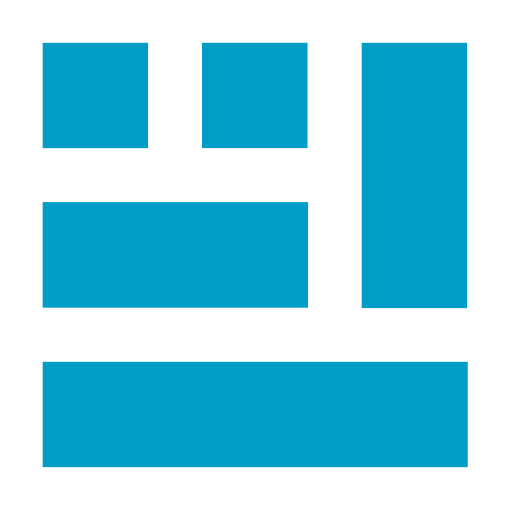
Ingestion
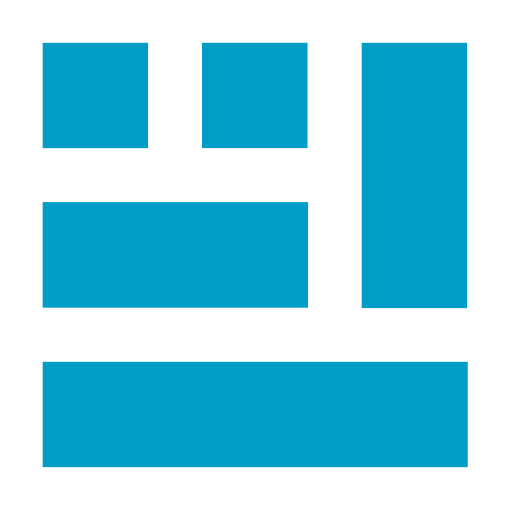
Native SQL support
CrateDB is a distributed database that implements native SQL and the PostgreSQL Wire Protocol. With CrateDB, you can easily query even complex and dynamic schemas in a familiar SQL format, without the need to learn custom languages. The massive parallel execution of queries ensures fast response times, making it ideal for handling ad-hoc queries across large datasets, including those commonly encountered in AI/ML applications.
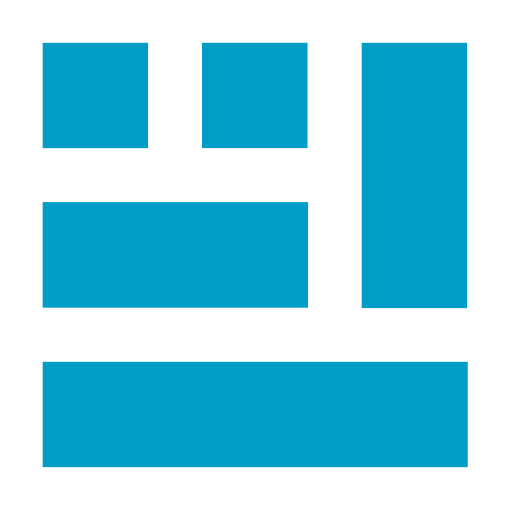
Ecosystem
CrateDB seamlessly integrates with your AI/ML stack (LangChain, MLflow, PyCaret ...) and analytics stack (Tableau, PowerBI ...) by leveraging the support of the PostgreSQL Wire Protocol.
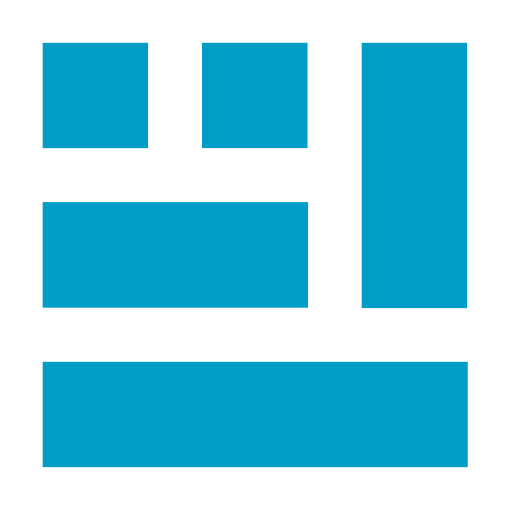
Reduced TCO
CrateDB offers a low Total Cost of Ownership (TCO) by eliminating the need to manage multiple systems. It seamlessly integrates your data, keeping your (meta-)data and vector representations aligned without the complexity of data synchronization processes. With its use of native SQL, CrateDB simplifies development and ensures compatibility with existing systems.
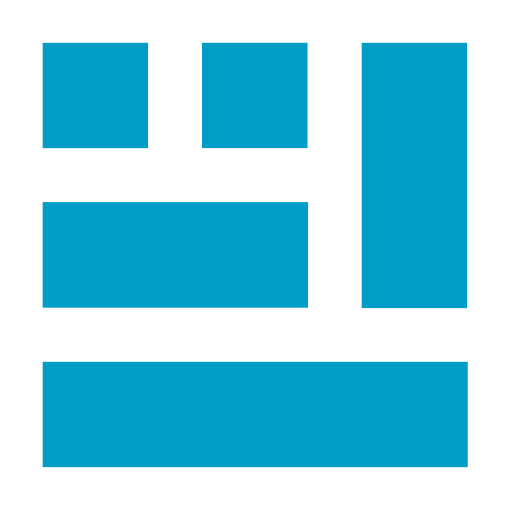
White Paper: How to Build AI-driven Knowledge Assistants with a Vector Store, LLMs and RAG Pipelines
This white paper explores how CrateDB provides a scalable platform to build Generative AI applications that cover the requirements of modern applications, such as AI-driven knowledge assistants. CrateDB is not just handling vectors, but also provides in a single storage engine a unique combination of all the data types needed for end-to-end applications, including RAG pipelines.
Demo – Harnessing CrateDB’s Multi-Model Capabilities for AI-Powered Applications
In this video, we explore the integration of CrateDB and PyCaret to detect anomalies in machine data, crucial for identifying potential failures or inefficiencies in technological systems. CrateDB's capability for handling large-scale data with ease pairs seamlessly with PyCaret's low-code approach to machine learning, offering a streamlined path to uncovering insights within vast datasets.
Demo: Building an AI Chatbot with CrateDB and LangChain
This video shows step by step how to build an AI-powered chatbot using LangChain to connect to the different LLMs and CrateDB to store embeddings and run similarity searches against them.
How to Build AI-driven Knowledge Assistants with a Vector Store, LLMs and RAG Pipelines
CrateDB at AI & Big Data Expo
CrateDB's VP Product shares his vision for the future with multi-model SQL databases and Large Language Models.
Webinar: Digital Twins & Gen AI on Azure
Explore how TGW, a global leader in logistics automation, digitally transformed warehouse operations using Azure. This session delves into the creation of automated warehouses and LLM-based internal Q&A system, answering general questions of employees, providing deep insights based on technical documentation and support tickets, and streamlining sales support.
User stories
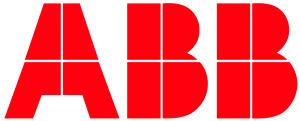
"Working with CrateDB brings positive outcomes. The ingestion and throughput have very good performance, with 1 million values/sec, the horizontal scalability where we can add as many nodes as we need and the automatic query distribution across the whole cluster"
Marko Sommarberg
Lead, Digital Strategy and Business Development at ABB
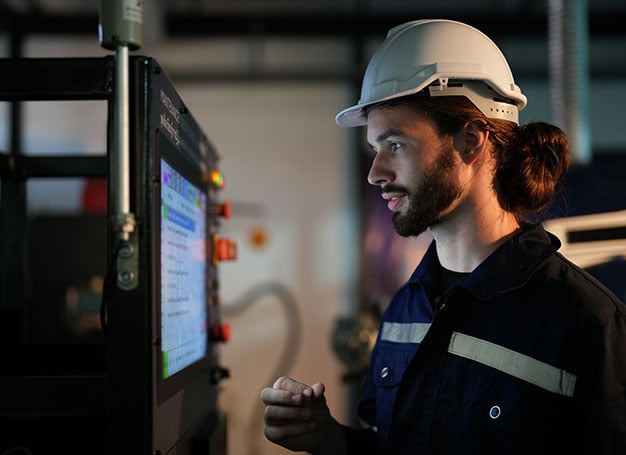
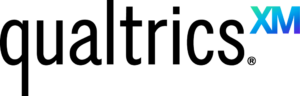
"CrateDB gives us ease of SQL combined with easy scaling, and real-time querying of full-text data."
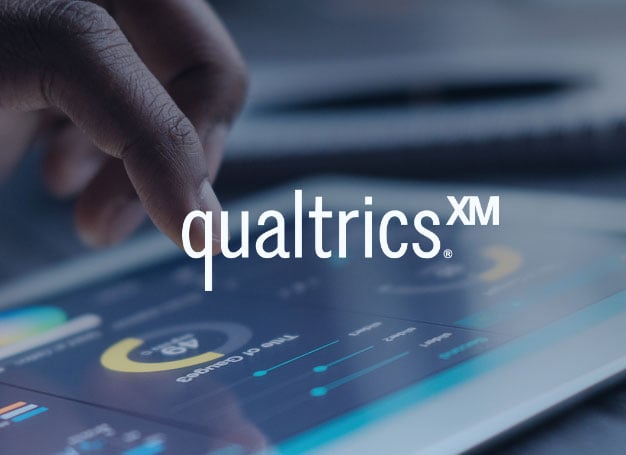
Related web pages
Related blog posts
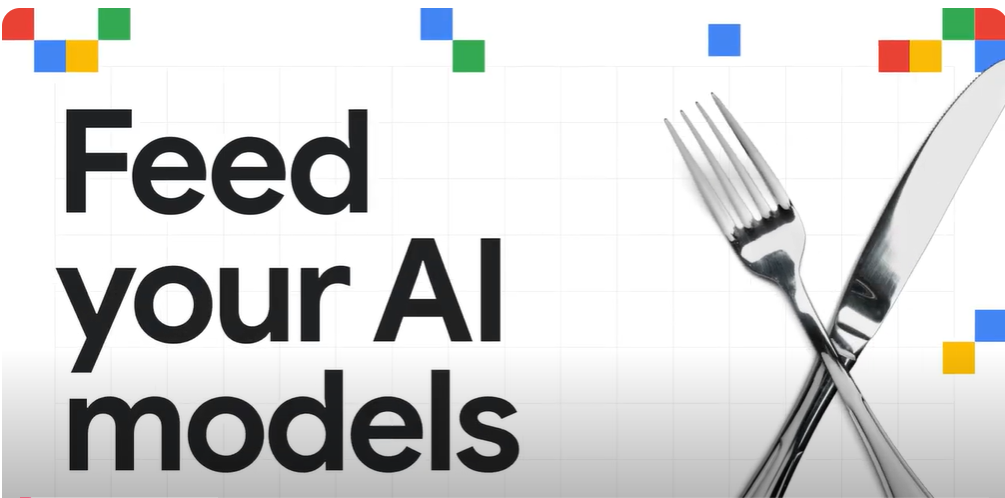
Video announcement with Google Cloud!
2024-10-25We are excited to get this new video announcement together with Google Cloud! Unleash the full potential of your data with CrateDB, the database for real-time analytics and hybrid search. CrateDB ...
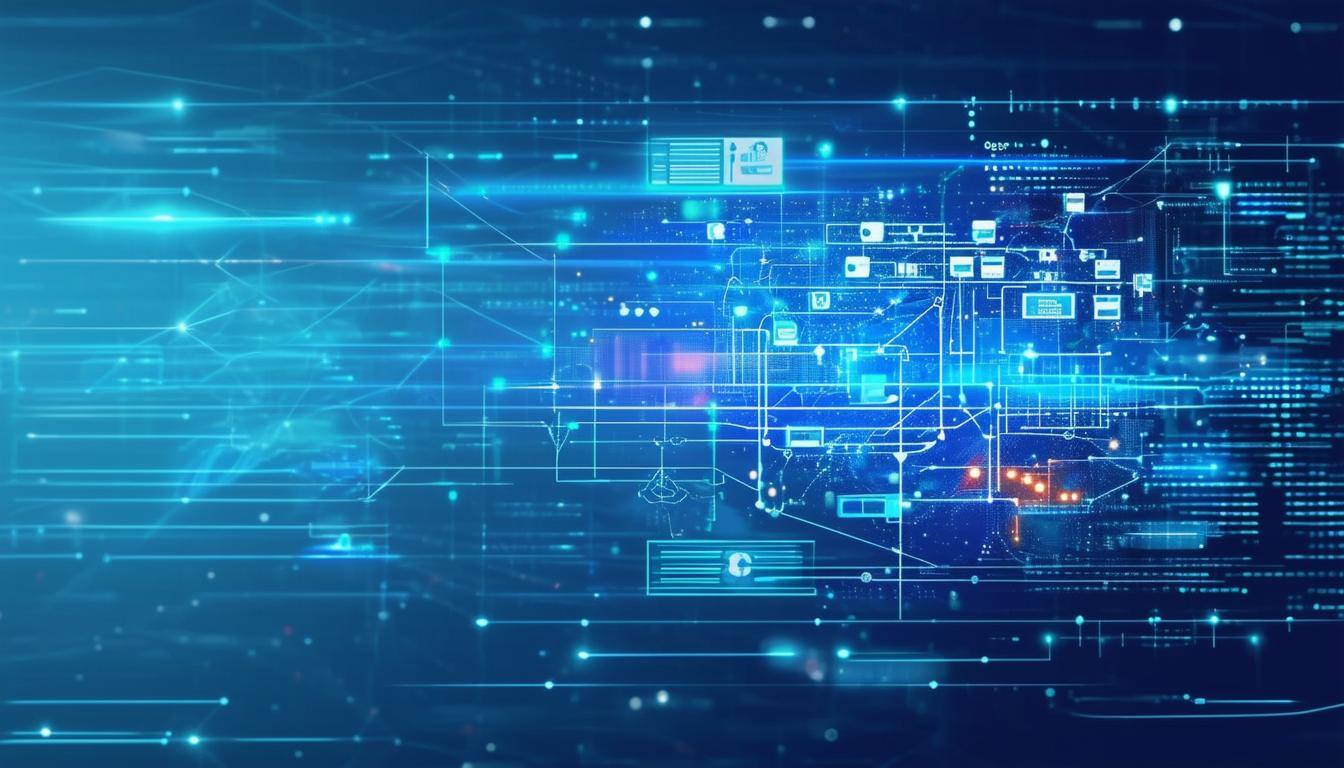
Unifying Data for Real-Time AI
2024-10-15Success in today's economy depends on the ability to make informed decisions in real-time based on huge volumes of diverse data.
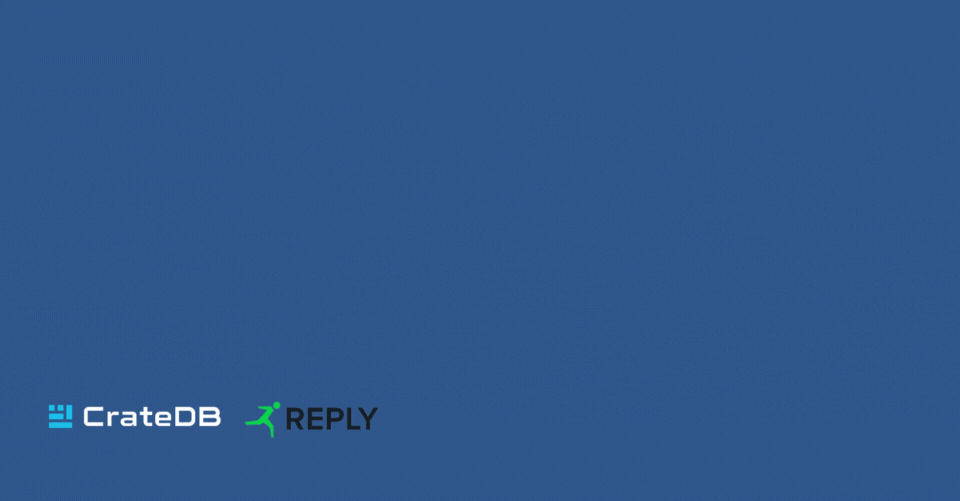
Data Challenges in Machine Learning and AI: An Interview with Machine Learning Reply
2024-03-13In a recent interview conducted by CrateDB with Ihor Shylo, Manager at Machine Learning Reply, a CrateDB partner, Ihor sheds light on the key data challenges companies are facing today while ...
Additional resources
Documentation
Documentation
Want to know more?
CrateDB is an open source distributed database designed for AI/ML use cases. It efficiently manages diverse data types and ensures real-time data accessibility for continuous model training and prediction. With vector storage and similarity search features, CrateDB unlocks new dimensions of efficiency in complex data analytics, pattern recognition, and AI. All of this is built on a scalable architecture that supports native SQL, facilitating streamlined querying and reducing system complexity. Whether in the cloud, on-premises, or at the Edge, CrateDB offers the flexibility and efficiency needed for all AI and ML operations.
FAQ
A database for AI and ML is a specialized storage system designed to store, manage, and retrieve machine learning models and large datasets used in AI applications. These databases are optimized for high performance and scalability, enabling efficient handling of the vast amounts of data and computational requirements needed for AI and machine learning tasks. They often support advanced features such as vector store support, advanced search capabilities, and integration with an ecosystem of machine learning frameworks and tools.
Some examples of databases for AI/ML include CrateDB, Pinecone, Zilliz, Weaviate, and DataStax. CrateDB is an open-source distributed database designed for AI/ML use cases, efficiently managing diverse data types and ensuring real-time data accessibility for continuous model training and prediction. Watch to learn how CrateDB's multi-model database approach enables real-time AI insights >
You should use an AI/ML database when you're working with large datasets, complex machine learning models, or applications that require real-time data analysis and prediction. These databases are useful when you need to perform frequent training and updating of models, ensuring that your AI solutions remain accurate and effective over time. With vector store and similarity search features, you can use CrateDB to perform complex data analytics and pattern recognition.
To choose the right AI/ML database for your needs, consider the size of your data, the required processing speed, and the scalability necessary for future growth. Assess the complexity of your machine learning models and ensure the database integrates well with your existing tools and technologies. Moreover, evaluate the costs of licensing, storage, and computational resources. CrateDB is an ideal choice for diverse needs due to its support for vector search and similarity search functionality, seamless integration with AI/ML stacks, and reduced total cost of ownership (TCO).
Unlike traditional databases, AI/ML databases are optimized for handling large volumes of diverse data types and performing high-speed analytical queries required for complex AI/ML tasks. CrateDB offers high scalability and integrates seamlessly with various AI and ML frameworks and tools.
- High Speed: They are optimized for rapid data ingestion, processing, and retrieval, which is crucial for time-sensitive AI applications. CrateDB delivers millisecond response times for complex queries, providing real-time insights and responsiveness.
- Scalability: Designed to scale horizontally or vertically, they can handle growing data volumes and increased computational loads without significant performance degradation. With its distributed shared-nothing architecture, CrateDB provides seamless scalability for hundreds of nodes with minimal operational effort.
- Efficient Storage: They offer advanced data compression and storage optimization techniques, reducing storage costs and improving data access speed. CrateDB optimizes data storage by partitioning and sharding large tables, ensuring balanced data distribution and improved query performance.
- Integrated Analytics: Many AI/ML databases support in-database analytics, allowing data scientists to build, train, and deploy models directly within the database environment, streamlining the workflow and reducing latency. CrateDB enhances this capability by providing advanced search functionalities and real-time querying, which enable data scientists to perform complex analyses and derive insights quickly within the same environment.