Geospatial Data
Geospatial data queries with SQL
Hyper-fast. Results in milliseconds.
/* Based on NYC taxi trips, this query lists the latest 5 trips starting
within 500m of the Times Square */
SELECT pickup_datetime AS "time",
trip_distance,
latitude(pickup_location) latitude,
longitude(pickup_location) longitude
FROM nyc_taxi.trips
WHERE distance('POINT(-73.985130 40.758896)'::GEO_POINT, pickup_location) < 500
AND pickup_datetime BETWEEN 1451602800000 AND 1483138800000
ORDER BY time DESC
LIMIT 5;
+---------------+---------------+--------------------+--------------------+
| time | trip_distance | latitude | longitude |
+---------------+---------------+--------------------+--------------------+
| 1467331195000 | 3.0 | 40.76302719116211 | -73.98388671875 |
| 1467331192000 | 1.8 | 40.761539459228516 | -73.98821258544922 |
| 1467331192000 | 4.59 | 40.75526809692383 | -73.98706817626953 |
| 1467331191000 | 3.6 | 40.75738525390625 | -73.99018096923828 |
| 1467331183000 | 1.7 | 40.75679016113281 | -73.98326110839844 |
+---------------+---------------+--------------------+--------------------+
/*
* Based on NYC taxi trips, this query calculates:
* - the distance of a given dropoff location to a fixed point of interest,
* partitioned in buckets of 50 meters each
* - the average trip distance in kilometers within that partition
* - the number of trips within that partition
*/
SELECT floor(
distance(t.dropoff_location, 'POINT(-73.984 40.758)') / 50
) * 50 AS point_distance,
avg(
distance(t.pickup_location, t.dropoff_location) / 1000.0
) AS trip_distance,
count(*) AS trips
FROM nyc_taxi.trips t
WHERE t.pickup_month = 1451606400000
GROUP BY 1
ORDER BY 1 ASC;
+----------------+---------------------------+--------+
| point_distance | trip_distance | trips |
+----------------+---------------------------+--------+
| 0 | 4.183248580260856 | 2258 |
| 50 | 3.5389173023149585 | 10891 |
| 100 | 2.973617177658283 | 23798 |
| 150 | 2.8757472450827946 | 41841 |
| 200 | 2.936262490313057 | 44880 |
| 250 | 2.901378357605571 | 44918 |
| 300 | 2.7757565505864257 | 49662 |
| 350 | 2.6239797352885708 | 57803 |
| 400 | 2.822579512102226 | 73603 |
| 450 | 2.5346381484458105 | 79051 |
| 500 | 2.4982467856661392 | 88705 |
| 550 | 2.613586563537282 | 93082 |
| 600 | 2.6103083633519786 | 98527 |
| 650 | 2.5381838408225286 | 113023 |
| 700 | 2.537684333523469 | 125640 |
| 750 | 2.5883437771402007 | 127099 |
| 800 | 2.617847584473263 | 119027 |
| 850 | 2.554740996422641 | 132532 |
| 900 | 2.499970010565691 | 164115 |
| 950 | 2.612569696294771 | 181091 |
| 1000 | 2.5512554347843093 | 152102 |
+----------------+---------------------------+--------+
/* Based on NYC taxi trips, this query returns the number
of trips per day with the region of the New York
Central Park as destination */
SELECT date_bin('24 hour'::interval, pickup_datetime, 0) AS day,
count(*) AS trips
FROM nyc_taxi.trips
WHERE within(
dropoff_location,
'POLYGON(
(-73.97308900174315 40.764422448981996,
-73.98192956265623 40.76812781417226,
-73.9584064734938 40.80087951931638,
-73.94982340464614 40.797240957024385,
-73.97308900174315 40.764422448981996)
)'
)
AND pickup_datetime BETWEEN 1417392000000 AND 1420070400000
GROUP BY 1
ORDER BY 1;
+---------------+-------+
| day | trips |
+---------------+-------+
| 1417392000000 | 5094 |
| 1417478400000 | 5580 |
| 1417564800000 | 5760 |
| 1417651200000 | 5970 |
| 1417737600000 | 5850 |
| 1417824000000 | 5472 |
| 1417910400000 | 5335 |
| 1417996800000 | 5573 |
| 1418083200000 | 5382 |
| 1418169600000 | 5484 |
| 1418256000000 | 6291 |
| 1418342400000 | 5953 |
| 1418428800000 | 5846 |
| 1418515200000 | 5177 |
| 1418601600000 | 5446 |
| 1418688000000 | 5639 |
| 1418774400000 | 6051 |
| 1418860800000 | 6161 |
| 1418947200000 | 6052 |
| 1419033600000 | 5695 |
| 1419120000000 | 4891 |
| 1419206400000 | 5050 |
| 1419292800000 | 4786 |
| 1419379200000 | 4637 |
| 1419465600000 | 2721 |
| 1419552000000 | 3789 |
| 1419638400000 | 4343 |
| 1419724800000 | 4048 |
| 1419811200000 | 4769 |
| 1419897600000 | 5126 |
| 1419984000000 | 5128 |
+---------------+-------+
Geospatial functions
With CrateDB geospatial functions, you can address these use cases easily:
- Spatial count: find how many records are located within a specific area, thanks to the within function.
- Spatial distance: find the closest record, thanks to the distance function.
- Spatial overlap: find whether two areas overlap, thanks to the within and intersects functions.
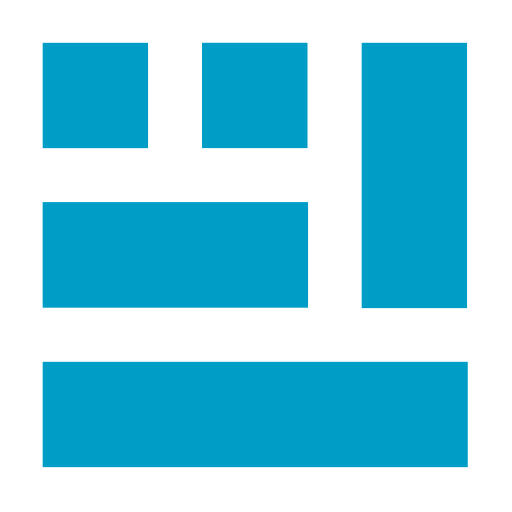
Geospatial data formats
Geopoints
CrateDB offers versatile support for geospatial data types. For storing geopoint data, there are two primary formats you can use in the database:
- Array Format: Geopoints can be represented as an array where the coordinates are in the format
[longitude, latitude]
. This array format provides a simple and straightforward way to store point locations. - WKT Format: CrateDB also supports the Well-Known Text (WKT) representation for geopoints. You can store geopoint data using the format:
POINT(longitude latitude)
. This notation provides a standardized way to represent geospatial data.
Geoshapes
For storing more complex geospatial shapes into the database, CrateDB offers the following formats:
- GeoJSON Format: This is a popular format for encoding a variety of geographical data structures. It’s versatile and can represent simple points, lines, and polygons as well as more complex shapes.
- WKT Formats: Apart from the POINT format, CrateDB supports a variety of Well-Known Text formats for geoshapes. Whether you’re storing polygons, lines, or multi-points, WKT offers a standard representation for each of these geospatial structures.
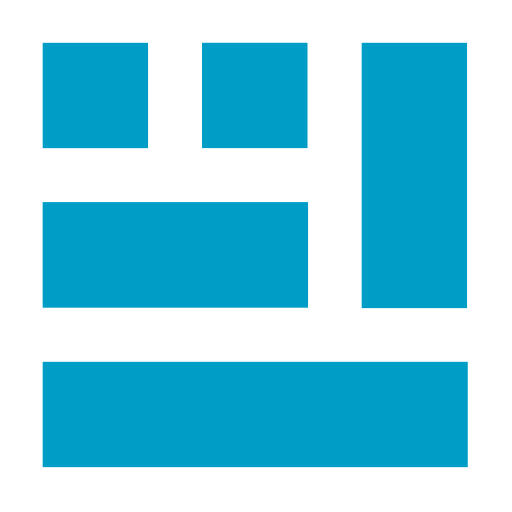
Interested?
Geospatial data has a lot of points and generates a lot of data, that’s why you need great scalability. With CrateDB, you get scalable SQL support for geospatial data types and functions for geospatial applications such as fleet tracking, mapping, location analytics.
Location is important for many machine data analysis. For this reason, CrateDB can store and query geographical information using the geo_point and geo_shape data types. You can control geographic index precision and resolution for faster query results, and also run exact queries with scalar functions like intersects, within, and distance.
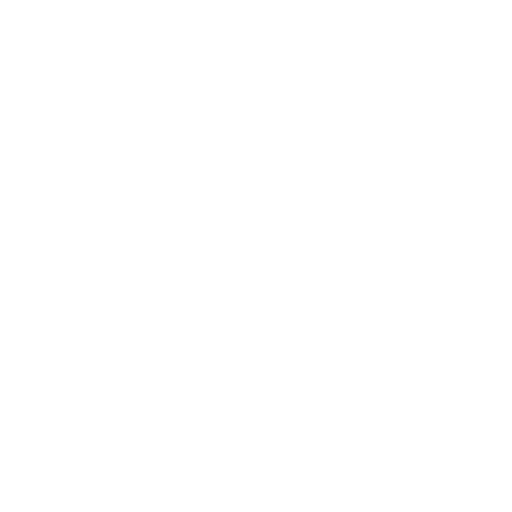
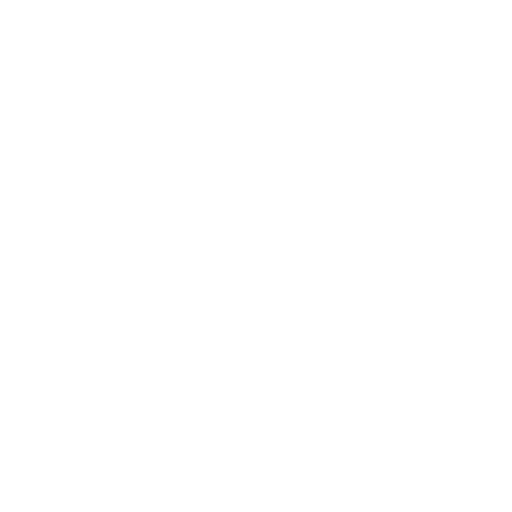
Other resources on geospatial data
Blog
Geometric Shapes Indexing with BKD-trees
Introduction of BKD-tree-based indexing that brings notable enhancements in query performance
Documentation
Geo data types
GEO_POINT, GEO_SHAPE
Documentation
Geo search
MATCH, intersects, within, distance
Blog
FAQ
Geospatial data represents specific geographical locations using latitude and longitude coordinates or text fields naming geographical areas like countries or states. This data is crucial for applications that require tracking and analyzing the location of people and objects. CrateDB supports the storage, analysis, and real-time tracking of geospatial data - all using SQL.
Spatial data encompasses various formats used to represent geographic locations and shapes. Common examples include arrays for simple point data and GeoJSON or WKT formats for more complex shapes. CrateDB supports these geospatial data types, offering flexibility and precision in storing and querying geospatial information.
Storing geospatial data efficiently requires a database with strong scalability due to the large volume of data points it generates. It should allow for the control of geographic index precision and resolution to enable faster query results and support exact queries with functions like intersects, within, and distance. CrateDB excels in this area by offering scalable SQL support for geospatial data types, including geo_point and geo_shape, making it ideal for geospatial applications.
The best databases for spatial data should offer advanced geospatial functions to handle spatial count, distance and overlap efficiently. Examples of databases optimized for spatial data include CrateDB, Amazon Aurora, Esri ArcGIS, and MariaDB. CrateDB supports robust geospatial capabilities, allowing for seamless integration and analysis of spatial data.