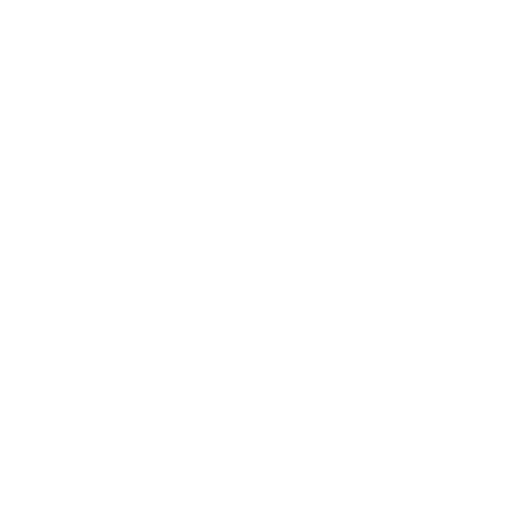
The extensive SQL querying capabilities of CrateDB include multiple functions:
Aggregations
CrateDB is built for fast aggregation using a columnar storage engine to speed up queries.
Count() function
The count(column) aggregate function simply returns the number of rows that match the query. When used with a column name as a parameter, it returns the count of rows where the specified column has a non-NULL value.
SELECT location, COUNT(*) FROM weather_data_json GROUP BY 1; +-------------+----------+---------------+ | count(name) | count(*) | date | +-------------+----------+---------------+ | 7 | 8 | 1373932800000 | | 4 | 4 | 308534400000 | | 1 | 1 | 1367366400000 | +-------------+----------+---------------+ SELECT 3 rows in set (... sec)
AVG() function
CrateDB employs the AVG function to calculate averages, such as temperature per location.
SELECT location, AVG(vals['humidity']) AS avg_humidity FROM weather_data_json GROUP BY 1; +---------------+--------------+ | location | avg_humidity | +---------------+--------------+ | San Francisco | 60.0 | | Sydney | 41.0 | +---------------+--------------+ SELECT 2 rows in set (0.003 sec)
MAX_BY and MIN_BY functions
For advanced queries, for example finding the highest and lowest temperatures with their corresponding times, CrateDB offers functions like MAX_BY and MIN_BY, demonstrating its facility to handle complex data aggregations.
SELECT location, MAX(ts) AS latest_reading, MAX_BY(vals['humidity'], ts) AS latest_humidity FROM weather_data_json GROUP BY 1; +---------------+----------------+-----------------+ | location | latest_reading | latest_humidity | +---------------+----------------+-----------------+ | San Francisco | 1705069860000 | 60.0 | | Sydney | 1705069860000 | 40.0 | +---------------+----------------+-----------------+ SELECT 2 rows in set (0.052 sec)
SELECT location, MIN(ts) AS earliest_reading, MIN_BY(vals['humidity'], ts) AS earliest_humidity FROM weather_data_json GROUP BY 1; +---------------+------------------+-------------------+ | location | earliest_reading | earliest_humidity | +---------------+------------------+-------------------+ | San Francisco | 1705069800000 | 60.0 | | Sydney | 1705069770000 | 41.0 | +---------------+------------------+-------------------+ SELECT 2 rows in set (0.005 sec)
Geometric_mean() function
The aggregate function geometric_mean(column) calculates the geometric mean, which is a type of average used for positive numbers. This function is applicable on all numeric types as well as timestamps and consistently returns double values.
SELECT location, GEOMETRIC_MEAN(vals['humidity']) AS geo_mean_humidity FROM weather_data_json GROUP BY 1; +---------------+--------------------+ | location | geo_mean_humidity | +---------------+--------------------+ | San Francisco | 59.999999999999986 | | Sydney | 40.991868306014425 | +---------------+--------------------+ SELECT 2 rows in set (0.052 sec)
Arbitrary() function
The arbitrary(column) function produces a single value from a specified column, however, it does not define which value is returned. The output type of this function matches the type of the parameter column used.
SELECT location, ARBITRARY(vals['humidity']) AS any_humidity FROM weather_data_json GROUP BY 1; +---------------+--------------+ | location | any_humidity | +---------------+--------------+ | San Francisco | 60.0 | | Sydney | 42.0 | +---------------+--------------+ SELECT 2 rows in set (0.003 sec)
You can find the list of all available aggregation functions in the documentation here.
Common Table Expressions (CTEs)
WITH hourly_stats AS ( SELECT location, DATE_TRUNC('hour', ts) AS ts_hour, AVG(vals['humidity']) AS avg_humidity, AVG(vals['temperature']) AS avg_temperature, AVG(vals['wind_speed']) AS avg_wind_speed FROM weather_data_json GROUP BY 1, 2 ) SELECT FROM hourly_stats s JOIN warning_thresholds t ON s.avg_wind_speed >= t.max_wind_speed WHERE ts_hour >= NOW() - '5 hours'::INTERVAL; +--------------+-------------+--------------+-----------------+----------------+----------------+ | location | ts_hour | avg_humidity | avg_temperature | avg_wind_speed | max_wind_speed | +--------------+-------------+--------------+-----------------+----------------+----------------+ |San Francisco |1705068000000| 60.0 | 17.5 | 35.6 | 35.0 | +--------------+-------------+--------------+-----------------+----------------+----------------+
Window Functions
For the list of the window functions available, check out the documentation here.
Interpolation of Missing Values
SELECT locations.location, s.ts, vals['temperature'] AS current_temperature, LAG(vals['temperature']) IGNORE NULLS OVER w AS prev_temperature, LEAD(vals['temperature']) IGNORE NULLS OVER w AS next_temperature FROM GENERATE_SERIES('2024-01-12 14:30:00', '2024-01-12 14:32:00', '1 minute'::INTERVAL) s("ts") CROSS JOIN UNNEST(['San Francisco', 'Sydney']) AS locationns("location") LEFT JOIN weather_data_json w ON locations.location = w.location AND w.ts = s.ts WINDOW w AS (PARTITION BY locations.location ORDER BY s.ts) ORDER BY 1, 2 DESC; +---------------+---------------+---------------------+------------------+------------------+ | location | ts | current_temperature | prev_temperature | next_temperature | +---------------+---------------+---------------------+------------------+------------------+ | San Francisco | 1705069920000 | NULL | 17.5 | NULL | | San Francisco | 1705069860000 | 17.5 | 16.0 | NULL | | San Francisco | 1705069800000 | 16.0 | NULL | 17.5 | | Sydney | 1705069920000 | NULL | 27.8 | 27.8 | | Sydney | 1705069860000 | 27.8 | 29.1 | NULL | | Sydney | 1705069800000 | 29.1 | NULL | 27.8 | +---------------+---------------+---------------------+------------------+------------------+ SELECT 6 rows in set (0.004 sec)
Moving Averages
SELECT ts, vals['humidity'] AS current_humidity, AVG(vals['humidity']) OVER last_2 AS avg_humidity_last_2_readings, AVG(vals['humidity']) OVER last_20 AS avg_humidity_last_20_readings FROM weather_data_json w WHERE location = 'San Francisco' WINDOW last_2 AS (PARTITION BY location ORDER BY ts ROWS BETWEEN 2 PRECEDING AND CURRENT ROW), last_20 AS (PARTITION BY location ORDER BY ts ROWS BETWEEN 20 PRECEDING AND CURRENT ROW) ORDER BY 1, 2 ASC LIMIT 5; +---------------+------------------+------------------------------+-------------------------------+ | ts | current_humidity | avg_humidity_last_2_readings | avg_humidity_last_20_readings | +---------------+------------------+------------------------------+-------------------------------+ | 1705069800000 | 60.0 | 60.0 | 60.0 | | 1705069860000 | 60.0 | 60.0 | 60.0 | | 1717686351524 | 20.0 | 46.666666666666664 | 46.666666666666664 | | 1717686353859 | 50.0 | 43.333333333333336 | a | | 1717686355629 | 55.0 | 41.666666666666664 | 49.0 | +---------------+------------------+------------------------------+-------------------------------+ SELECT 5 rows in set (0.054 sec)
Joins
SELECT w.ts, w.location, s.software_version, s.geo_location, w.vals['temperature'] AS temperature, w.vals['humidity'] AS humidity FROM UNNEST(['San Francisco', 'Sydney']) AS locations("location") LEFT JOIN weather_data_json w USING (location) LEFT JOIN weather_station s ON s.location = locations.location; +---------------+---------------+----------------+-------------------------------------------+-----------+--------+ | ts | location |software_version| geo_location |temperature|humidity| +---------------+---------------+----------------+-------------------------------------------+-----------+--------+ | 1705069800000 | San Francisco | C-5.rev2 | [122.23999997600913, 37.45999999344349] | 16.0 | 60.0 | | 1705069860000 | San Francisco | C-5.rev2 | [122.23999997600913, 37.45999999344349] | 17.5 | 60.0 | | 1705069800000 | Sydney | A-1 | [151.11999998800457, -33.520000032149255] | 29.1 | 42.0 | | 1705069770000 | Sydney | A-1 | [151.11999998800457, -33.520000032149255] | 28.5 | 41.0 | | 1705069860000 | Sydney | A-1 | [151.11999998800457, -33.520000032149255] | 27.8 | 40.0 | +---------------+---------------+----------------+-------------------------------------------+-----------+--------+ SELECT 5 rows in set (0.003 sec)
Additionally, CrateDB provides a host of other useful functions that are essential for data analysis:
- Data binning: The DATE_BIN function is useful for grouping data by time-based intervals, e.g., every 10 seconds. It is also a handy tool for harmonizing non-equidistant events to correlate them to equidistant events.
- Sampling of the dataset: In some use cases, it might be necessary to sample the dataset. This can easily be achieved with a modulo operator on the internal doc id attribute.
- User Defined Functions: CrateDB allows for domain-specific logic to be put into user-defined functions, making it reusable in an easy and consistent manner. For example, we can calculate the temperature in Fahrenheit from degrees Celsius using a dedicated function.
CREATE FUNCTION to_fahrenheit (c DOUBLE) RETURNS DOUBLE LANGUAGE JAVASCRIPT AS 'function to_fahrenheit(c) { return (c * (9/5)) + 32; }';